Artificial Intelligence: Four questions for underground infrastructure professionals
Publicerad: 10 augusti, 2020
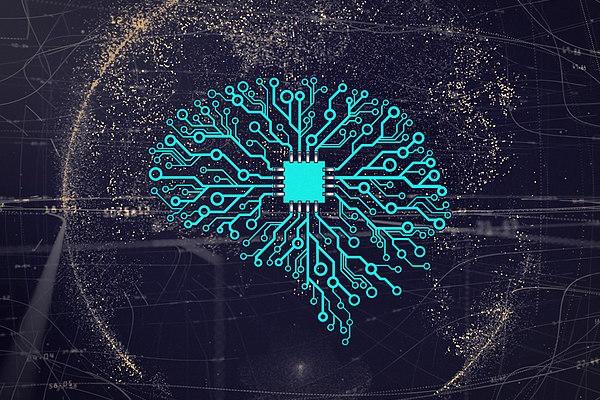
Wikimedia Commons/mikemacmarketing /photo on flickr
Artificial Intelligence is increasingly being applied to some of the most critical aspects of our daily lives. In the underground infrastructure space – particularly wastewater, drinking water and stormwater asset management – AI will have a major impact over the next several years on many process, workflows and deliverables.
NEED TO KNOW RIGHT NOW?
When people hear about AI, it’s likely a lot comes up: robots coming to replace peoples’ jobs, chess geniuses losing to computers, utopian and dystopian sci-fi scenarios, being amazed (and scared) when our faces are automatically recognised in pictures on social media, etc. While all that is valid, the term ‘AI’ is basically just the automation of tasks involving characteristics of human intelligence such as recognition of objects or sounds, or in complex problem-solving and analysis.
Many people also use the terms ‘machine learning’ or ‘deep learning’ interchangeably with AI, but that’s problematic, because technically AI can be achieved without machine learning. However, that method, which involves manually hard-coding routines with specific instructions for the millions of possible scenarios and decisions involved in most complex tasks is just not practical.
Not to mention, this kind of program wouldn’t be able to improve its performance on its own without constant human interventions. So, instead of a manually programmed AI, software engineers use machine learning as a way of training an algorithm so that it learns for itself how to fulfill a specific objective, and this happens by exposing the algorithm to various examples that enable it to adjust itself to make improvements over time.
To distinguish ‘machine learning’ from ‘deep learning’, we could go down a rabbit hole, but for the sake of accuracy let’s just say that deep learning is a subset of machine learning, except deep learning involves multiple layers of machine learning algorithms that when integrated, constitute a ‘neural network’, also referred to as an artificial neural network or convolutional neural networks.
TASK AUTOMATION OR BIG DATA?
We are seeing two primary areas in underground infrastructure where AI is making a big difference. In task automation, for example, we are seeing companies using AI to enable automation ofnhighly repeatable, rote tasks, such as the navigation of robotic inspection drones to collect data in underground infrastructure, particularly pipelines.
Another example of task automation is computer vision, which is when an AI gains a high-level understanding of objects and/or conditions from digital images or videos, such as CCTV data collected from sewer and storm pipeline inspections. Currently, several companies have developed computer vision software that automatically recognises defects and features in pipes, and assists in generating condition assessment reports, at a significantly faster rate compared to when these tasks are carried out manually, to a significantly higher degree of accuracy and consistency and while costing far less.
In the realm of Big Data, the main focus is on ways AI can assist and support human decision makers like asset managers and engineers through analytics. We are seeing more and more offerings seeking to comb through otherwise unmanageable or disparate datasets to extrapolate useful insights, predictions or descriptions.
This is already having a huge impact in drinking water, wastewater flow monitoring and even infectious disease tracking such as COVID-19 (which can be detected in sewer discharges), as well as in stormwater modelling and asset management in general.
One of the main applications of AI-based analytics in underground infrastructure asset management is in calculating risk (an asset’s “likelihood of failure” x “asset’s criticality”) scenarios. Another is use of AI-based analytics to drive decision making in asset rehabilitation and renewal strategies.
WHICH TYPE(S) OF AI WILL BE MOST RELEVANT TO ME?
If you are wondering whether or not AI will affect your job or people in your industry subset, then you’re asking the wrong question. The answer is yes, AI will most definitely have an impact on you and your work and will most likely occur by the end of this decade. A more useful question might instead be: what specific aspects of my work, and relatedly, what kind(s) of AI will I be interacting with?
The first places to look are the aspects of a job which involve highly repetitive/rote tasks (automation), as opposed to aspects which might involve critical thinking, historical trends and external events (analytics). If your job involves data collection, ask yourself which tasks are manually performed versus tasks that may already be automated, and then start to wonder if those tasks are not already automated, why not?
Not every task currently being manually performed is inevitably going to be automated but it’s a valid place to look, and there’s a fairly low technology hurdle to overcome to automate certain tasks you engage in, then expect for it to become automated.
What also makes a difference is for one to be able to look at workflows in your organisation and in your industry, and to start to distinguish the information being captured or processed in these workflows, and whether it’s quantitative or qualitative information, and importantly, how is this information being used? For what kind of purpose: descriptive, predictive or prescriptive?
IS AI INEVITABLE?
Predicting the future is easy, but accurately predicting the future is not. Anyone willing to research what it was like for inventors and pioneers who introduced many of our now-ubiquitous technologies can find predictions and responses of their contemporaries which are now, in hindsight, laughable. Here are a just a few:
• 1876: “The Americans have need of the telephone, but we do not. We have plenty of messenger boys.” – William Preece, British Post Office.
• 1903: “The horse is here to stay but the automobile is only a novelty – a fad.” – President of a Michigan bank, in denying an investment loan to Henry Ford’s partner.
• 1946: “Television won’t be able to hold on to any market it captures after the first six months. People will soon get tired of staring at a plywood box every night.” – Darryl Zanuck, 20th Century Fox.
Speaking of accurate predictions, let’s understand that in each of the above examples, the technology already existed, but the question of “will this be adopted on a large scale?” had yet to be settled. Just because something exists, doesn’t mean it will necessarily be used.
In today’s times, technology investors are constantly challenging inventors, who can prove that something can be done, to demonstrate why it should be done.
Analysts in the AI space are noting the rate of innovation and improvement is outpacing Moore’s 'law' (an approximate doubling every two years in processor capabilities). Stanford’s AI Index 2019 has even found “the time required to train a network on cloud infrastructure for supervised image recognition fell from about three hours in October 2017 to about 88 seconds in July 2019.”
While current and upcoming AI innovations are sure to be impressive to machine learning insiders, it does not necessarily mean a given breakthrough will translate into the larger workforce or make a real difference in whatever industry it’s intended for.
As an underground construction professional who has focused on trenchless technologies and pipeline robotics for the last 13 years, I have seen far too many novel innovations, each with their own measurable value proposition, fail to take off.
With many of these, the promoters of the technology might have even been diligent to quantify the extent of the value added with their innovation, to publish case studies of results for early adopters, even to the point where some (like myself ) would consider it to be a no brainer scenario that would surely make a huge impact on my industry, only to see (years later) these companies or product offerings fail to take hold, for whatever reason (strategic marketing mistakes, bad business practices or bad luck, etc).
As underground infrastructure professionals seek to navigate the uncertain future caused by the COVID-19 global pandemic, where we are seeing water and wastewater utilities (in the US at least) experience an unprecedented loss in revenue on the magnitude of tens of billions of dollars, and who have already been dealing with a wave of retirements among industry professionals, and the loss of that institutional knowledge, there absolutely will be an increased demand for solutions that enable organisations to do more with less, which is a classic problem AI can solve.
Nevertheless, it will be essential for those bringing AI solutions to the marketplace to communicate responsibly to the stakeholders in the industry, and in a way that addresses many of the valid concerns about the workforce disruptions caused or perceived by AI.